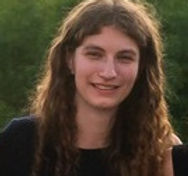
Helene Huts
KU Leuven (KUL)
Academic and professional background, key areas of expertise, and research interests
MSc. Biomedical Engineering. Currently working on a PhD at KU Leuven with focus on the estimation of individual treatment effects with causal machine learning.
What is your role within the AI-PROGNOSIS project, and how do your expertise and skills contribute to achieving its objectives?
Member of the research team for task 3-6: Medication response predictive modelling. Causal machine learning models can uncover individual treatment effects for this task.
What future opportunities do you see for your field in similar healthcare projects?
General causal machine learning methods can be applied to investigate any disease and any treatment. The application potential is very large.
What do you see as the long-term potential of the AI-PROGNOSIS project in advancing AI-driven healthcare solutions?
This project can be a foundation for many useful AI tools in Parkinson’s disease and pave the way for similar projects in other medical fields.
From a clinical perspective, how do you think the AI-PROGNOSIS tools will influence the diagnosis, prognosis, and treatment of Parkinson’s disease?
Personalised predictions of treatment effect can support clinicians to make more targeted use of medication. This increases effectiveness and helps avoid unnecessary costs and side-effects.
How do you envision AI specifically supporting the AI-PROGNOSIS project, and what direct benefits do you anticipate from its integration?
AI methods can help uncover valuable, complex patterns in data that cannot be detected with more standard techniques.
How are user research and co-creation part of the AI-PROGNOSIS project, and what benefits do you expect from working together in these ways?
Close collaboration with clinicians ensures that AI tools are properly adapted to clinical needs.